Seasonal forecast of two-metre temperature and precipitation in Tanzania: A hybrid cluster and point-by-point machine learning approach
Authors
Camille Sansonnet, Michiel Schaeffer, and Florent Baarsch
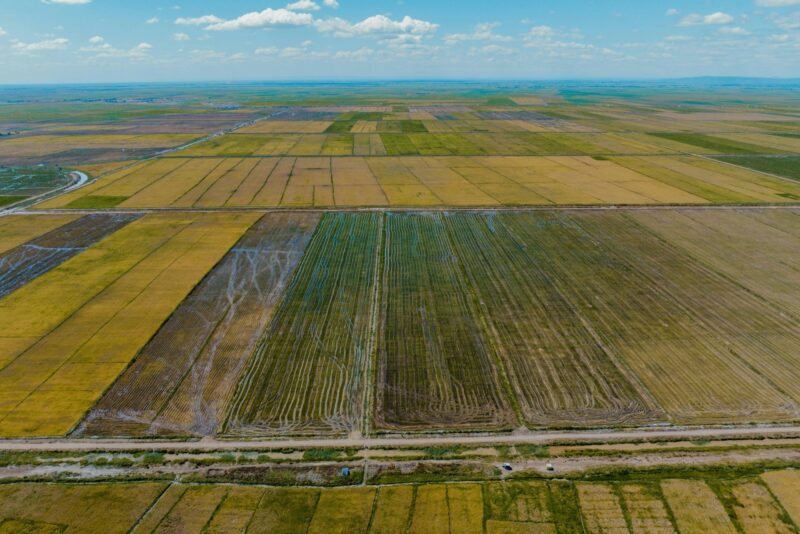
Seasonal forecasting of atmospheric parameters is important in various fields including agriculture. Machine learning makes it possible to forecast at lower computational costs than dynamic models. This advantage is even more important in certain regions of the world.
In seasonal weather forecasting, the exact location within a study area determines the relationship between local predicted variables and global predictors. Both dynamic models and machine learning approaches that define models for single grid points can determine these relationships with high spatial granularity, but at high computational cost.
To avoid the latter, clustering of predicted variables is often used in machine learning approaches, which however sacrifices geographical resolution. In this paper, we present a machine learning approach that is a hybrid between gridpoint and cluster-based approaches (finres_S2S). This approach preserves geographical resolution, but at low computational cost, and is tested for monthly 2-m temperature (2T) and precipitation (TP) in Tanzania and for a lead time of up to 6 months.
The finres_S2S approach has a number of advantages over both the cluster and point approaches, including that of obtaining good results compared to a dynamic model. We find that the dominant predictors for the application area are associated with El Niño–Southern Oscillation, the Madden–Julian oscillation, and the Indian Ocean dipole.
The hybrid model developed here (finres_S2S) allows seasonal forecasting with a spatial resolution equal to that of ERA5 data and a low cost in calculation time. The seasonal forecast scores outperform those of ECMWF. The development of the use of machine learning in seasonal forecasting seems to provide a complementary response to dynamic models, and research efforts in this direction should be pursued.