Hydrologic interpretation of machine learning models for 10-daily streamflow simulation in climate sensitive upper Indus catchments
Authors
Haris Mushtaq, Taimoor Akhtar, Muhammad Zia ur Rahman Hashmi, Amjad Masood and Fahad Saeed
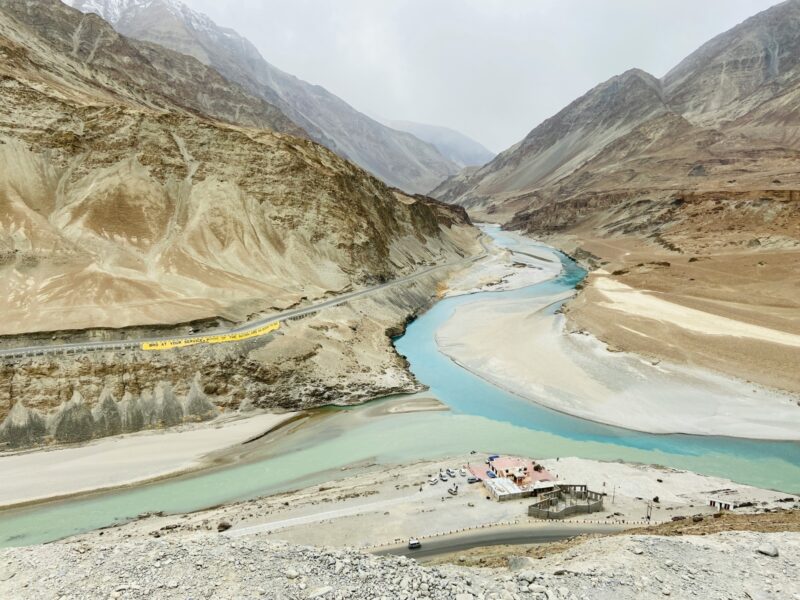
Machine learning for hydrologic modeling has seen significant recent development and has been suggested as a valuable augmentation to physical hydrological modeling, especially in data-scarce catchments.
In Pakistan, surface water flows predominantly originate from the transboundary Upper Indus sub-catchments of Chenab, Jhelum, Indus, and Kabul rivers. These catchments have large drainage areas, climate-driven streamflows, high variations in elevation, and limited streamflow gauge coverage. Hence, using machine learning models for data-driven river flow modeling may be well-suited for these catchments.
However, hydrologic interpretability of machine learning models is important for the practical use of such models for these catchments. Thus, the current study besides evaluating the potential of three machine learning models (XGBOOST, Classification and Regression Trees(CART), and RandomForest) for streamflow simulation also focused on the hydrologic interpretation of machine learning models using SHapley Additive exPlananations (SHAP).
All of these models performed well and the range of R2 and Nasche-Efficiency for all three models lies between 0.61 to 0.90. Moreover, SHAP correctly identified minimum temperature as the most critical feature in glacier-fed Indus and Chenab catchments. It also provides logical insights into interactions between minimum temperature and precipitation for the Indus and Chenab catchment. The findings of this study strongly illustrate the usefulness of SHAP analysis in interpreting the behavior of data-scarce high-elevation climate-sensitive catchments using tree-based machine learning models.